
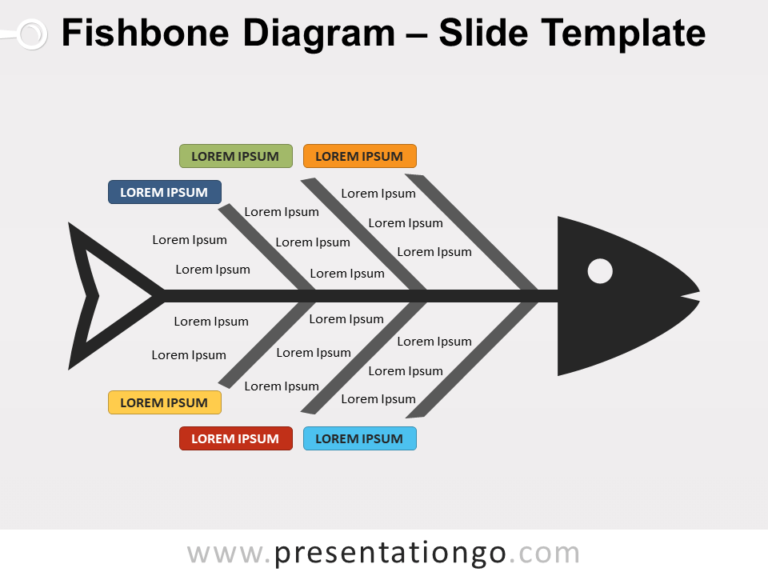
It works only in coordination with the primary cookie. Records the default button state of the corresponding category & the status of CCPA. The cookie is used to store the user consent for the cookies in the category "Performance". This cookie is set by GDPR Cookie Consent plugin. The cookie is used to store the user consent for the cookies in the category "Other. The cookies is used to store the user consent for the cookies in the category "Necessary". The cookie is set by GDPR cookie consent to record the user consent for the cookies in the category "Functional". The cookie is used to store the user consent for the cookies in the category "Analytics". Set by the GDPR Cookie Consent plugin, this cookie is used to record the user consent for the cookies in the "Advertisement" category. These cookies ensure basic functionalities and security features of the website, anonymously. Necessary cookies are absolutely essential for the website to function properly.
#Creator of fishbone diagram iso#
The boxed red words represent the groupings (ex: Tools, Employees, Data sources) and the “boxless” red words represent the main root causes (ex: Free text fields, No training, No ISO adoption). Here is a fishbone diagram example covering the different root causes of bad addresses. Add root sub-causes: Identify why each cause happens and add them as sub-causes. If they pertain to multiple categories, add them in each one.Ĥ. Add root causes: Brainstorm the main reasons for bad data quality and point them to the category they pertain to.
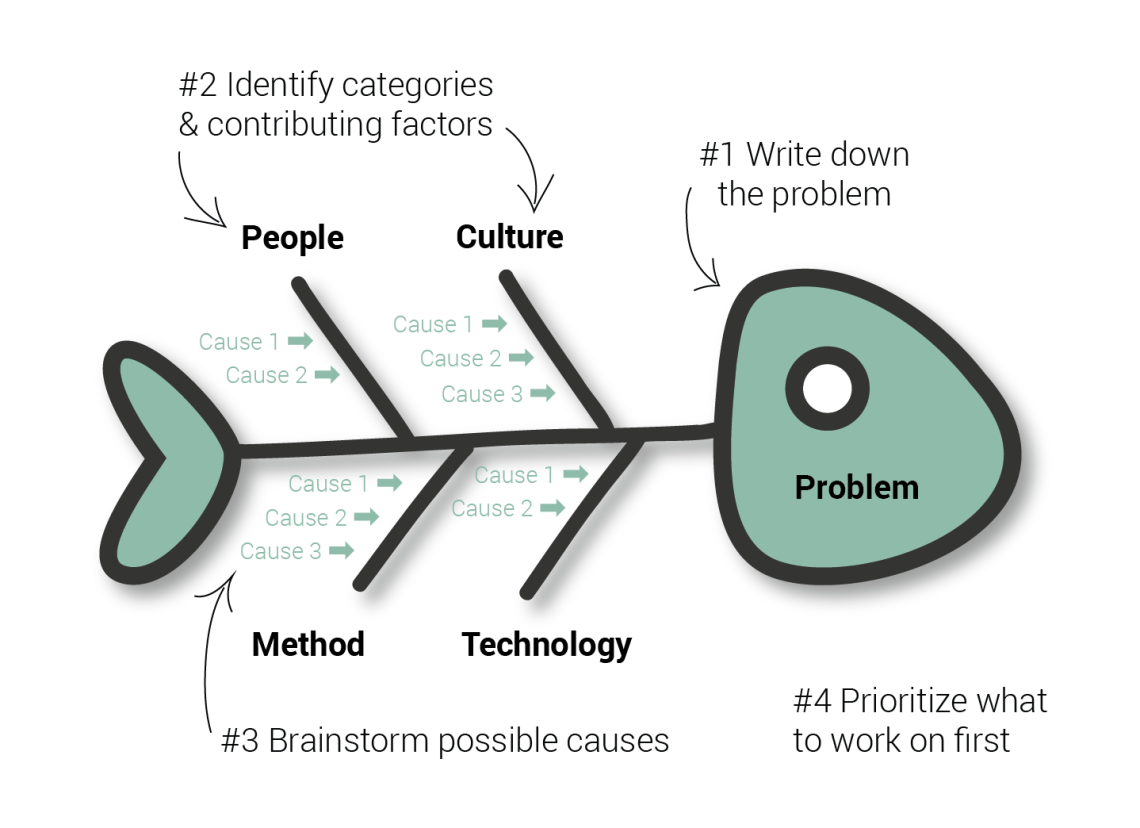
If you need a helping hand, start with the following: tools, employees, processes, standards, data sources. Point each category into the fishbone spine.ģ. Determine the categories: Brainstorm the main categories of the root causes. the head of he fish). Draw a horizontal arrow from the left of the diagram to the right, pointing it to the data quality issue. Note it on the right of the diagram (i.e. State the data quality issue: This is the issue for which you will determine the root causes. Here are the 4 myths about data quality everyone thinks are true.ġ. Interrelationships between causes are not easily identifiableĪvoid the pitfalls of bad data quality.Complex issues might yield a lot of causes which might become visually cluttering.Good visualization for presenting issues to stakeholders.Allows you to see all causes simultaneously.Quickly see if the root cause is found multiple times in the same or different causal tree.A highly visual brainstorming tool which can spark further examples of root causes.If the team’s thinking is not going towards a root cause.While conducting a workshop to identify the possible causes of the issue.The main issue is recorded on the right side of the page because traditional Japanese script reads down a vertical column from right to left across the page. The basic concept was first used in the 1920, but the actual fishbone diagram was popularized in the 1960s by Kaoru Ishikawa who pioneered quality management processes in the Kawasaki Shipyards. The end result looks like a fishbone, hence its name. The diagram focuses on the multiple root causes for one data quality issue. Each root cause or reason for bad data quality is added to the diagram and grouped into categories to identify and classify these causes. Ishikawa diagram, herringbone diagram, cause-and-effect diagrams, or Fishikawa Description: Tool used to identify the possible causes for an issue, in our case a data quality issue. To get you started, I’m offering a free fishbone diagram template focused on bad address data, at the end of this article.
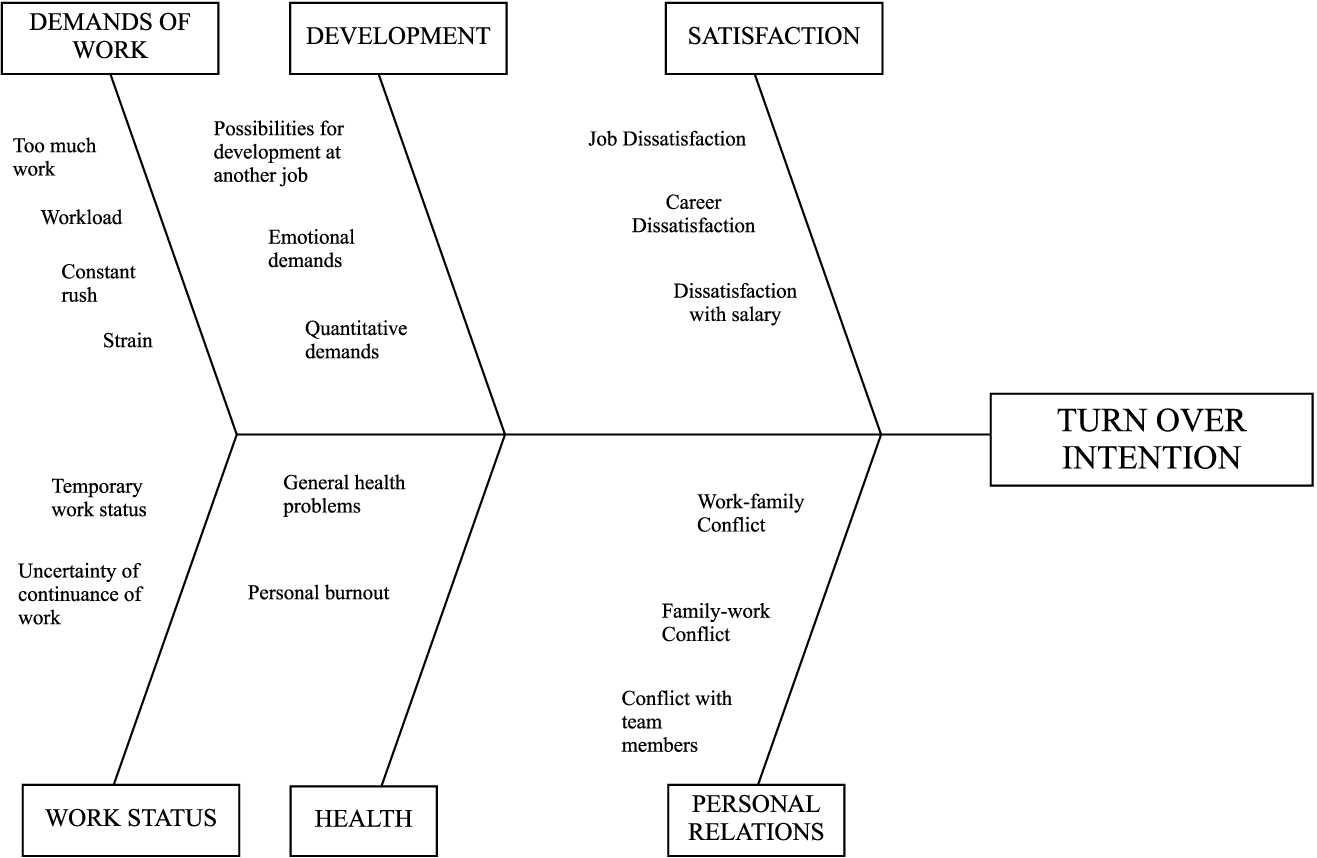
This one will focus on the fishbone diagram, in the context of data quality. There are many data quality root cause analysis techniques you can use and I will start covering the most important ones in future articles. Otherwise you will keep spinning your wheels and using your resources to constantly correct the issue, but never addressing the cause(s). As part of a sustainable data quality program, you need to identify the issues of your bad data.
